Alaukik Saxena successfully defended his dissertation
Congratulations to Alaukik Saxena for the successful defense of his dissertation on "Machine learning workflows for automatic analysis of atom probe tomography data".
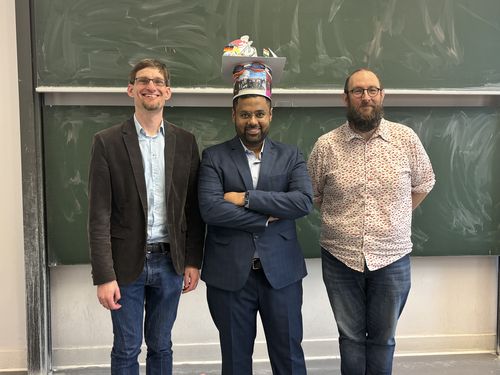
Alaukik Saxena successfully defended his dissertation on "Machine learning workflows for automatic analysis of atom probe tomography data".
The summary of his thesis is as follows:
This thesis focuses on enhancing the analysis of 3D microstructures in materials using machine learning techniques in the context of atom probe tomography (APT). APT provides detailed 3D mapping of atomic positions within materials, but analyzing these large datasets has traditionally been labor-intensive. To address this, the thesis introduces several machine learning-based workflows that automate the process, emphasizing robustness and reproducibility.
The research begins with a multi-stage, unsupervised machine learning approach to segment chemical domains within APT data, using clustering techniques to identify distinct phases and microstructural features. It then develops a supervised machine learning pipeline to analyze complex planar subdomains like grain boundaries, assessing their composition and geometry.
For linear features like dislocations, a skeletonization method is applied to reduce complex 3D structures into simpler forms for detailed analysis. Crystallographic orientation analysis is then performed to link these dislocations to the material's properties. Additionally, the study uses SOAP descriptors with neural networks to detect ordered domains in noisy APT data.
Overall, this thesis presents machine learning models that significantly improve the efficiency and accuracy of analyzing microstructures in APT datasets, advancing the understanding of how material structures influence their properties.