Ann-Kathrin Edrich successfully defended her dissertation
Congratulations to Ann-Kathrin Edrich for the successful defense of her dissertation on "Machine learning based landslide susceptibility assessment: Challenges of study design and opportunities for early warnings".
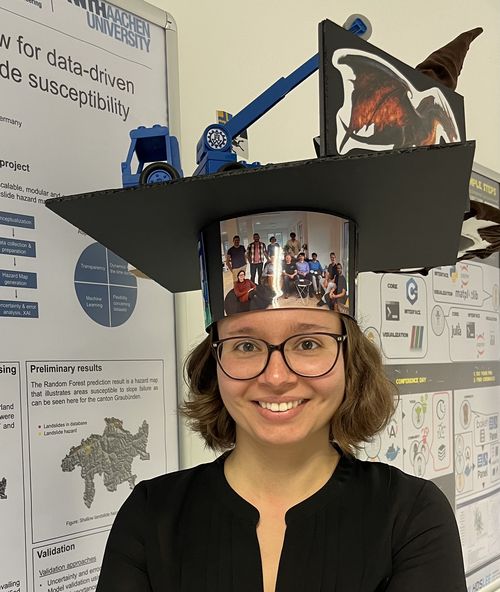
Ann-Kathrin Edrich successfully defended her dissertation on "Machine learning based landslide susceptibility assessment: Challenges of study design and opportunities for early warnings".
The abstract of her thesis is as follows:
"Landslides endanger people, infrastructure, and the environment worldwide. Therefore, it is important to assess the spatial and temporal hazards posed by landslides to develop and implement targeted mitigation measures. Landslide susceptibility and hazard mapping, conducted for this purpose, is a dynamic field of research. In recent years, machine learning has become increasingly important due to the availability of public datasets and increased computing power.
Most machine learning-based hazard mapping studies follow the same steps: (1) study design, (2) data search, (3) data pre-processing, (4) generation of input datasets for the algorithm, and (5) map generation. This process needs to be accompanied by a comprehensive validation strategy to ensure reliable and transparent results. Given the similarity of steps across susceptibility and hazard mapping studies, the development of software with a generic implementation of these steps offers a time-saving and standardised option for susceptibility and hazard mapping.
In recent years, several software packages have been released that use machine learning for mapping. These packages differ greatly in terms of functionality. However, none is notable for its transparency and reproducibility. Due to the growing demand for these properties in scientific studies and software, SHIRE (Susceptibility and Hazard MappIng fRamEwork) is presented in this thesis. SHIRE includes data pre-processing, input data generation, and mapping using a Random Forest model. During development, special attention was paid to explainability, reproducibility, and transparency of the process, as well as the interim and final results. SHIRE is published as Open Access and can be easily adapted to study-specific requirements due to its modular structure.
In this thesis, SHIRE is applied to a test case to investigate and discuss the challenges in study design and the opportunities for landslide early warning systems based on machine learning. The process of susceptibility and hazard mapping involves subjective decisions that significantly impact the quality of the final result. Many of these decisions are made during the initial study design phase, yet their detailed influence on the final outcome is often overlooked in the literature. To address this gap, this paper includes a sensitivity analysis focusing on various aspects affecting the design of the training dataset for the algorithm. The analysis is conducted using the test case of shallow landslide susceptibility in the Swiss canton of Grisons. It highlights the substantial influence of feature selection, the sampling strategy of non-landslide locations, and the representativeness of the training data.
Furthermore, incorporating a temporal component into susceptibility mapping enables the use of machine learning for early warning systems. Predicting the temporal occurrence of landslides requires integrating precipitation, their main time-dependent trigger, into the mapping process. However, accurately representing precipitation values for non-landslide areas poses challenges due to the inherent absence of timestamps. This paper presents approaches to generate representative precipitation values for non-landslide locations as well as their comparison and validation using the test case mentioned above. Finally, the lessons learned and the results obtained are summarised to provide recommendations and insights for future studies."