Mario Rüttgers successfully defended his dissertation
Congratulations to Mario Rüttgers for the successful defense of his dissertation on "Automated Surgery Planning for Improved Nasal Breathing".
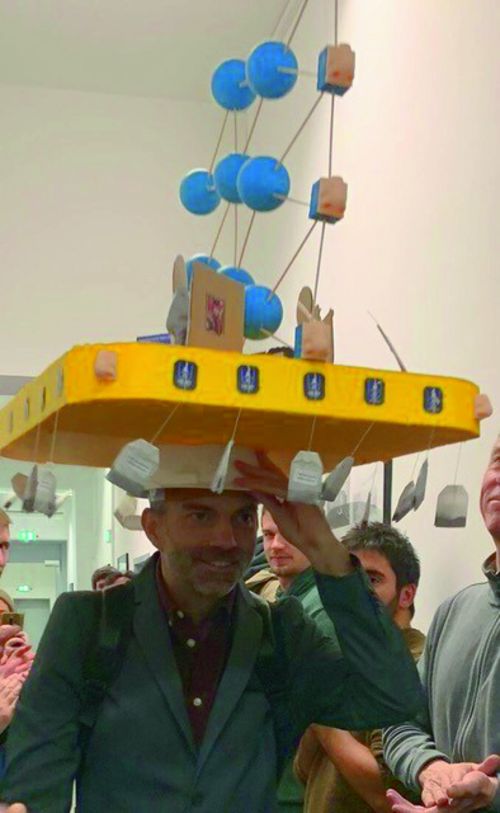
Mario Rüttgers successfully defended his thesis "Automated Surgery Planning for Improved Nasal Breathing". In his thesis, he investigated fluid dynamics to plan automatically surgeries to improve nasal breathing. The abstract of his thesis is as follows:
"Computational fluid dynamics is widely employed in various disciplines to investigate flow fields. However, depending on the complexity of a problem or the desired accuracy, setting up and employing such simulations can be time consuming and costly. Recently, machine learning techniques have shown great potential in automated workflows for flow applications. In this work, the potential for combining machine learning and flow simulations is studied for an example from life sciences. The example focuses on an automated data-processing pipeline for planning surgical interventions in the respiratory tract based on computed tomography images.
First, the pipeline standardizes medical images with low resolutions by employing a super-resolution network that outputs sufficiently resolved images for reliable flow simulations.Of course, such a step is only needed if the resolution of the original recordings is too low. The necessity of the step is highlighted by comparing flow simulations based on fine medical data with simulations based on coarse data, interpolated data, and data generated by the super-resolution network.
Second, the nasal cavity surface is extracted and subdivided into different boundaries with the help of convolutional neural networks. Based in this subdivision, boundary conditions can be assigned automatically to each boundary when performing the simulations. This step makes manual pre-processing obsolete and eases the usage of flow solvers in clinical environments for users without knowledge about preparing numerical simulations. The surfaces are then used to generate a grid and compute the flow field with the multiphysics-Aerodynamisches Institut Aachen flow solver.
Third, an object detection network is trained to localize and classify nasal obstructions in computed tomography recordings. Such a detection assists a surgeon in finding pathologies efficiently. Furthermore, it is shown, how the inclusion of computational fluid dynamics data to the network input improves detections.
Finally, a reinforcement learning algorithm is coupled to the flow solver to find those modifications of the nasal airway that yield favorable breathing conditions. After each modification, the algorithm receives feedback in terms of fluid mechanical quantities that are extracted from simulation results.
It is shown how the algorithm can help a surgeon to plan a surgery by suggesting interventions that are backed up by fluid mechanical quantities. Additionally, the importance of different fluid mechanical parameters can be adjusted according to a surgeon's or patient's preferences by the choice of weighting factors.
Overall, the findings of this thesis reveal the enormous potential of the developed pipeline for a widespread usage in clinical environments to improve the success rate of surgical interventions in otorhinolaryngology. It lays the foundation for future studies aiming at reducing the number of follow up surgeries that must often be conducted to satisfy a patient's breathing conditions."