Robin Hilgers successfully defended his dissertation
Congratulations to Robin Hilgers for the successful defense of his dissertation on "Prediction of Magnetic Materials for Energy and Information Combining Data-Analytics and First-Principles Theory".
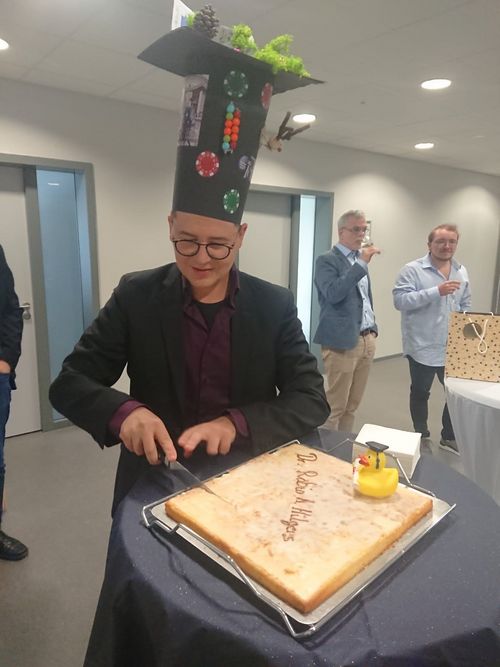
Robin Hilgers successfully defended his dissertation on "Prediction of Magnetic Materials for Energy and Information Combining Data-Analytics and First-Principles Theory".
The summary of his thesis is as follows:
The thesis explores the role of magnetic materials in reducing energy consumption in data storage and processing, focusing on how materials science simulations can aid in a green IT transformation. It presents workflows and best practices for high-throughput screening of magnetic materials for next-generation technologies. The study emphasizes the application of data science and machine learning (ML) to predict complex material properties, such as the critical temperature of Heusler alloys, achieving high prediction accuracy with ML models.
Additionally, the thesis examines the ML-based prediction of near half-metallicity in Heusler compounds, identifying new compounds with these properties. It highlights how ML can complement traditional physical models by extracting insights from data, even without prior knowledge of the physics involved. Moreover, large-scale simulations of ultrathin magnetic films were conducted to identify materials suitable for spintronics applications. A new scalable method was developed, significantly improving computational efficiency and reducing the time required for simulations. The thesis concludes by discussing the benefits, limitations, and implementation of ML and explainable artificial intelligence in materials science.