One of the grand challenges in life science is the understanding of biological systems as a whole and over multiple scales based on huge data sets that are nowadays available (genetic, molecular, and structural data bases) or can be generated in high throughput experiments (‘omics’ methods).
Relevant applications range from targeted design in synthetic biology (especially in biotechnology, e.g., for antibiotics production) over the diagnosis and treatment of systemic diseases (cancer, neurodegenerative diseases) up to the understanding of the human brain. Thus, systems biology, systems neuroscience, and systems medicine have experienced a tremendous increase of knowledge about biochemical and cellular networks over the recent decades. Driven by modern high-throughput omics and other massively parallel data acquisition methods, holistic models of complex biological systems like biotechnological production organisms, cellular tissues or neuronal networks are now constructed. However, the enormous amount of heterogeneous and multi-scale data poses intrinsic problems for data science.
Moreover, there are still too many white spots on the system map. For this reason, modern systems approaches require the combination of first principle and/or mechanistic modeling with methods of statistical data analysis, data bridging and prediction. The challenge of data integration from various measurement modalities and scales, the efficient assimilation, processing and quality assurance of input data for modeling, the close coupling of model based data evaluation procedures with experimentation and the efficient hypothesis generation from a complex overall systems phenotype are still unsolved problems.
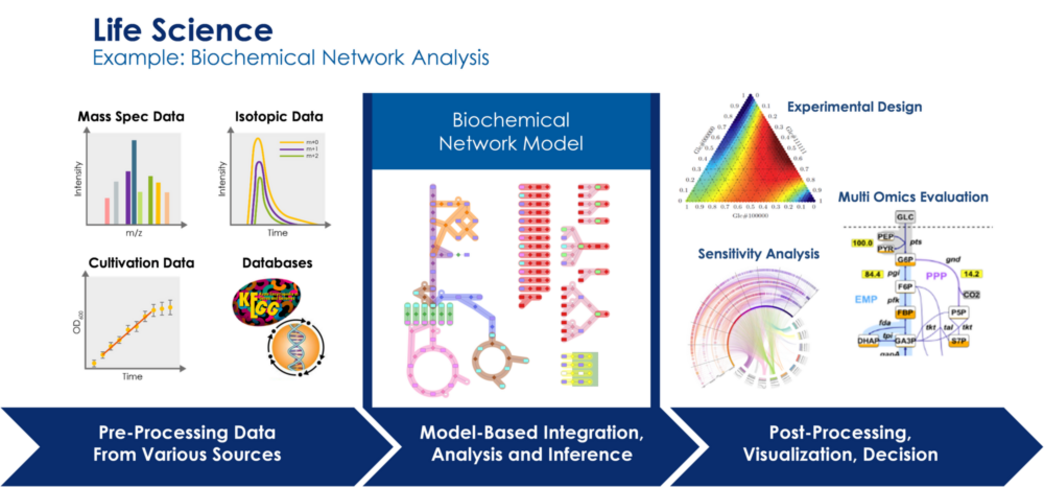